(RAI)volutionizing Master Data
Many of us have lived through the pain of failed master data projects. Sitting in evangelical sales meetings being told we’re going to solve all of our company's issues if we just get mastered data. Turning to despair a year in when we’ve only just successfully debated what a master customer or product looks like. Burning the midnight oil realizing our vision can’t be completed without stripping away huge amounts of valuable data to fit it into a single application.
Going back to the 1980’s, long before our data ecosystems evolved to include dozens, hundreds, and thousands of disparate applications, companies have been trying to create federated “golden records” of our most common domains. We typically start with customer, product, location, and then add countless variations of accompanying data. For many reasons we’ll address later, 76% of master data management (MDM) projects fail, according to a Gartner study.
One of the last product MDM projects I worked on was an attempt to master all products across each of a Fortune 100’s sub brands and tie them all into a centralized data model. Their goal was to understand product hierarchies across dozens of subsidiary companies to better inform marketing, product development, and sales data. For the first time, they would have a single, comprehensive point of view to manage their entire product line and its attached workloads.
For months we met with key stakeholders across the various brands, collecting data and debating how all these brands' data models would feed into the master data system. Each had a different view of what master data was, had different definitions for their data model, and it became impossible to create one unified view that each sub brand could agree on.
The final product workshop was chaotic. Over 100 representatives from the different business teams and 80 internal architects all sat in a room and walked through product workflows and data model reviews. Each person provided conflicting feedback, and the project was deadlocked. Without monumental and heroic effort from a single source of authority, the project would never succeed while attempting to boil the ocean.
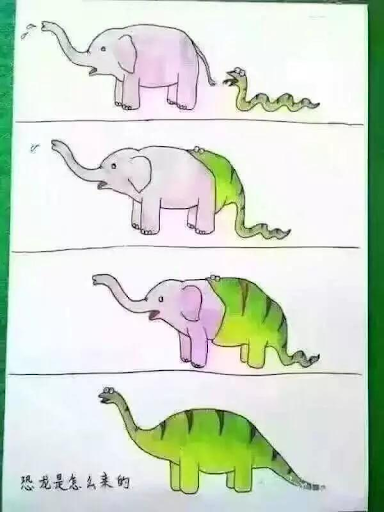
Most master data projects fail because we’ve relied on monolithic MDM platforms, and therefore centralized, highly federated approaches to creating master data. The MDM concept is strong. The benefits and uses of master data can provide value to those corporations who successfully implement it. However, we need to fundamentally change our approach to implementation. For that, we need tooling that allows for organic master data.
Organic master data is the idea that we are able to model our data domains based on how different teams use that data, and do so piece by piece. These teams share their “best version” of data domains into a single development layer, and we are able to combine it with other teams’ “best versions” to solve multiple business problems. Building master data in the piecemeal way causes minimal disruptions while providing constant and extended value.
Changing the Approach
For MDM projects to meet the high growth and proliferation of data we’ve seen in the 2010’s on, we must let go of the idea of federation and embrace the organic way that expertise grows in corporations. Like the populations of cities, data is typically stored in fiefdoms or silos across corporations. Nations have risen and fallen due to their natural resources, geography, climates, and populations required to support their infrastructure. The same can be said for data silos.
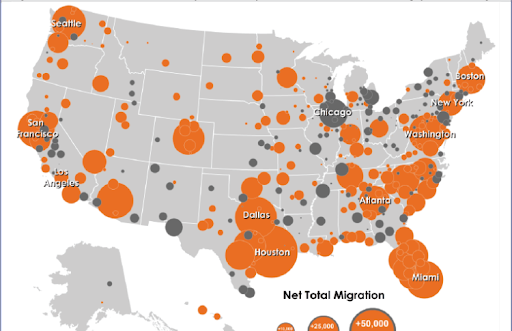
Different teams build up infrastructure based on the data they manage and interact with. Headcount growth is assigned to these teams based on the value of their data. Tribal knowledge forms, and domain expertise becomes embedded in application code. Each silo creates golden records for the data domains they have expertise in. Again, like expertise gained by a nation’s populations, this data domain expertise will overlap. As corporations, we should leverage all of our team’s expertise as they’ve defined it, and make it usable to all.
When we take the traditional approach to master data, we lock representatives from each of these expert silos in meeting rooms for weeks, months - and the worst I’ve seen - years at a time. All to debate and determine what each master domain and golden record will look like, where the data will come from, and how we’ll merge similar domains together.
All to fit into the monolith. This is why three quarters of these projects fail. We go against the way expertise is naturally formed and applied to our data. We strip away expertise, or try to shove it all into applications that can’t handle the load.
RelationalAI lets you model small pieces of truth without taking on your whole data ecosystem. We use our declarative language Rel to model and combine smaller pieces of master data together, to get away from “boiling the ocean”.
Welcome to the (RAI)volution
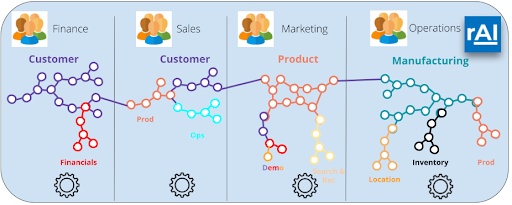
RelationalAI provides a data-centric solution for app development, with a relational Knowledge Graph at its core. To meet the needs of organic master data, we provide a development platform that completes the modern data stack.
The different fiefdoms across your organization will be able to model their data domains, and apply application logic directly against that model. This logic includes data validations, matching, taxonomy references, metadata, and governance policies, as well as logic used in analytics and AI/ML workloads, all to express, execute, and share knowledge within an organization.
This allows each team to provide their versions of golden records. Each team's store of data domains that we model, and all the application code embedded, becomes shareable, queryable, and linked. We send in missionaries, not mercenaries, to stitch your data together, bringing everyone to the table in a collaborative rather than a combative approach.
As more teams run their data-centric workloads in RAI, we add to the collective intelligence of our corporations. For end users, our teams can now easily access incorporated expert knowledge from other groups into their data apps. Our developers choose the most relevant master data to feed into UIs or reports that are use-case specific, and highly flexible.
Using RAI as your development platform enables you to start with pieces of expertise, the sum of which provide a master data store that powers data-centric use cases.
Are you planning out a master data or 360 project? Are you in the midst of an implementation and want to pivot and ensure its success? Let RelationalAI show you our solutions and make you the hero your company needs!