Human in the Loop Enrichment of Product Graphs with Probabilistic Soft Logic
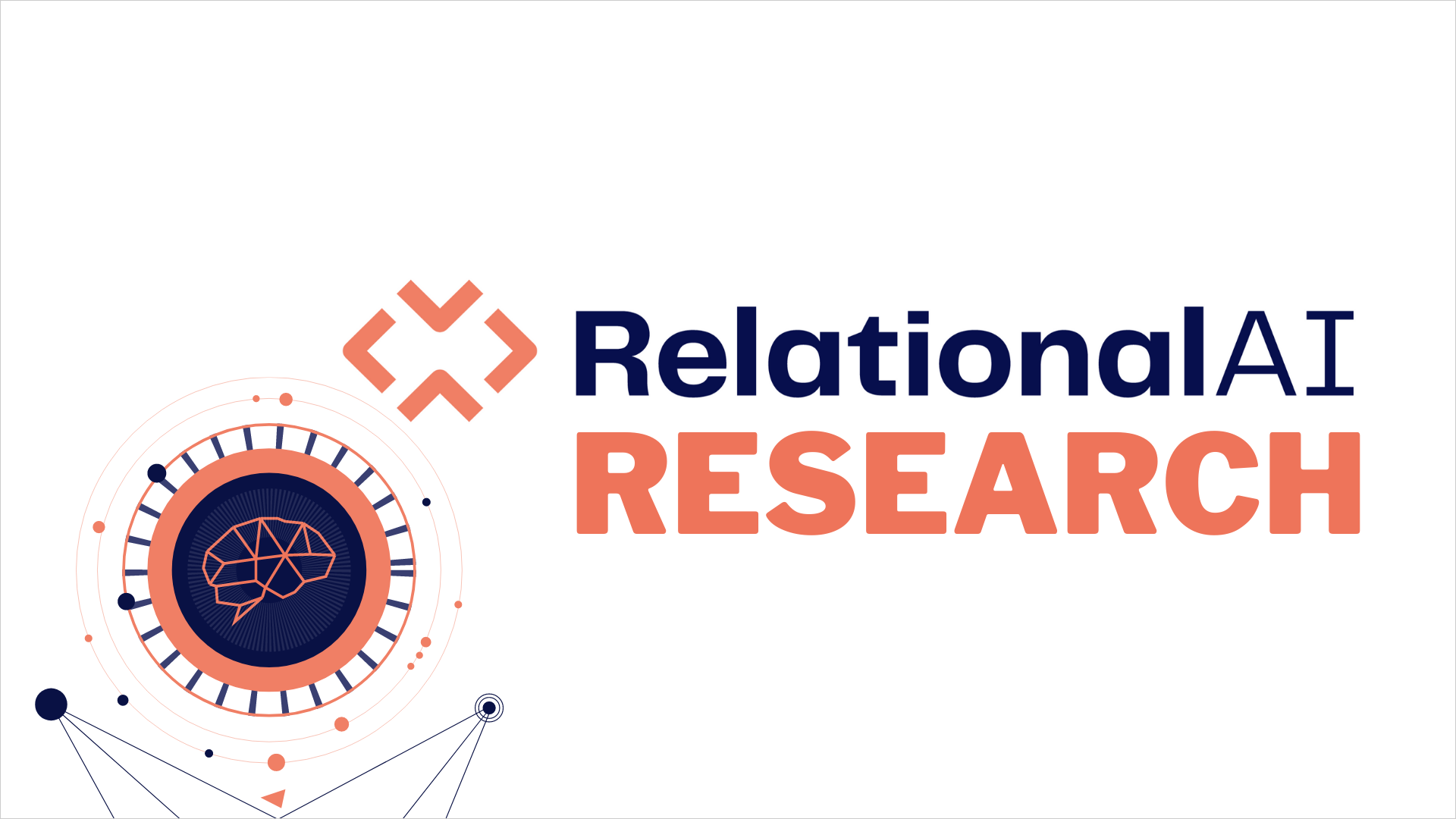
Product graphs have emerged as a powerful tool for online retailers to enhance product semantic search, catalog navigation, and recommendations. Our work complements existing efforts on product graph creation, by enabling field experts to directly control the graph completion process.
Authors: Marouene Sfar Gandoura, Zografoula Vagena, Nikolaos Vasiloglou. 2020.
In KDD 2020 Workshop on Knowledge Graphs and E-commerce (KGE, KDD ‘20).
Product graphs have emerged as a powerful tool for online retailers to enhance product semantic search, catalog navigation, and recommendations. Their versatility stems from the fact that they can uniformly store and represent different relationships between products, their attributes, concepts or abstractions etc, in an actionable form. Such information may come from many, heterogeneous, disparate, and mostly unstructured data sources, rendering the product graph creation task a major undertaking. Our work complements existing efforts on product graph creation, by enabling field experts to directly control the graph completion process.
Read the PDF: Human in the Loop Enrichment of Product Graphs with Probabilistic Soft Logic (opens in a new tab)
Related Posts
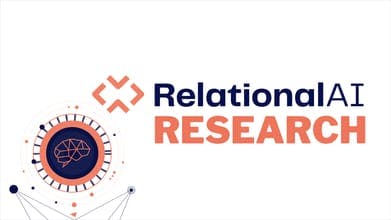
Learning Models over Relational Data using Sparse Tensors and Functional Dependencies
Integrated solutions for analytics over relational databases are of great practical importance as they avoid the costly repeated loop data scientists have to deal with on a daily basis: select features from data residing in relational databases using feature extraction queries involving joins, projections, and aggregations; export the training dataset defined by such queries; convert this dataset into the format of an external learning tool; and train the desired model using this tool.
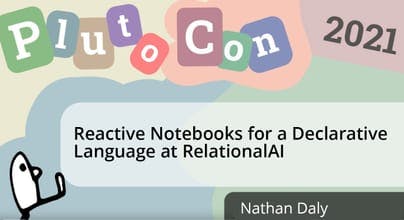
PlutoCon 2021 - Reactive Notebooks
At RelationalAI, we believe relational knowledge graphs are the foundation for future data-centric systems, and we are excited to demo the reactive notebook environment we built for working with knowledge graphs here with you!