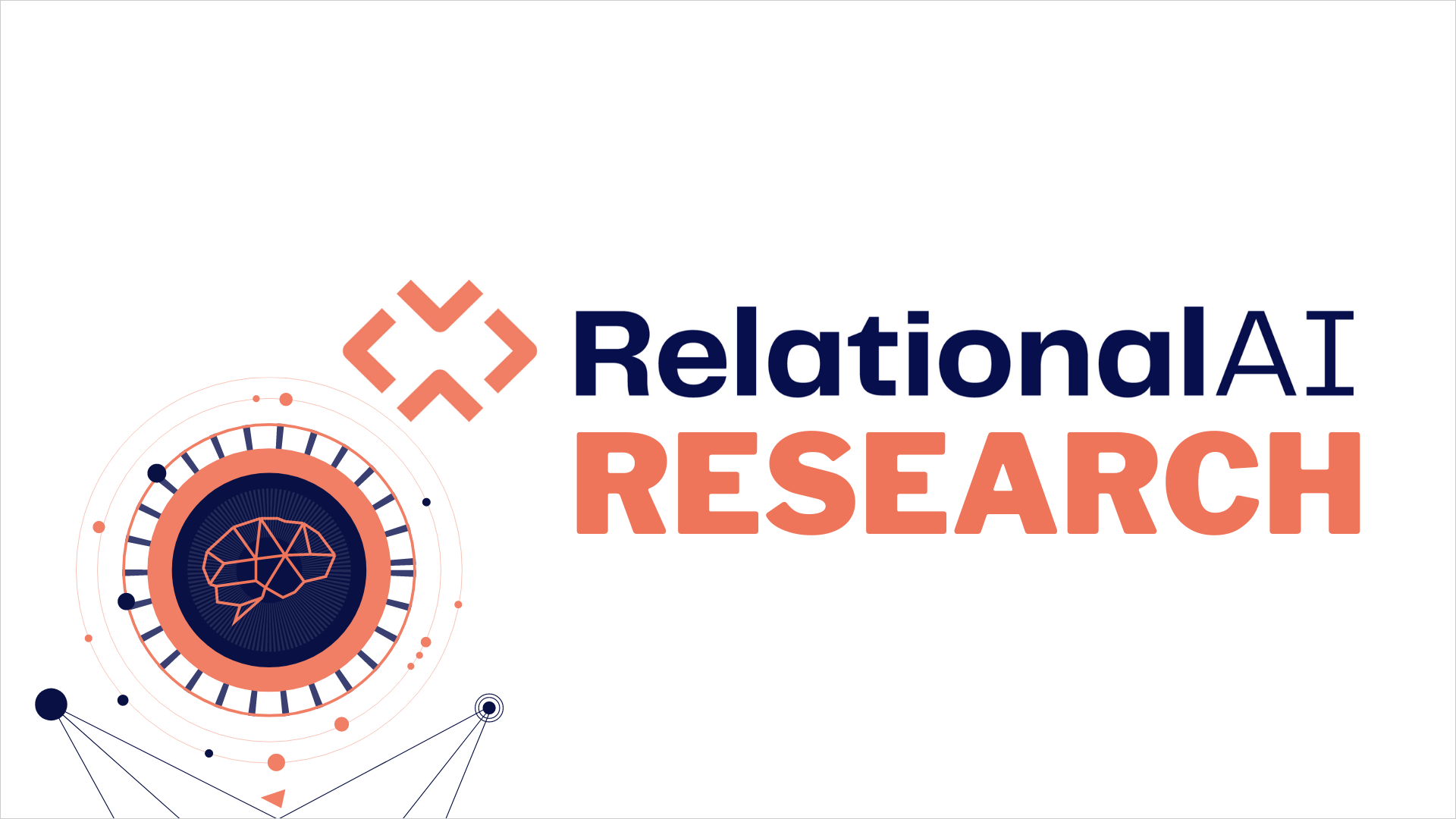
Optimizing Training Data for Image Classifiers
In this paper, we propose a robust method for outlier removal to improve the performance for image classification. Increasing the size of training data does not necessarily raise prediction accuracy, due to instances that may be poor representatives of their respective classes.
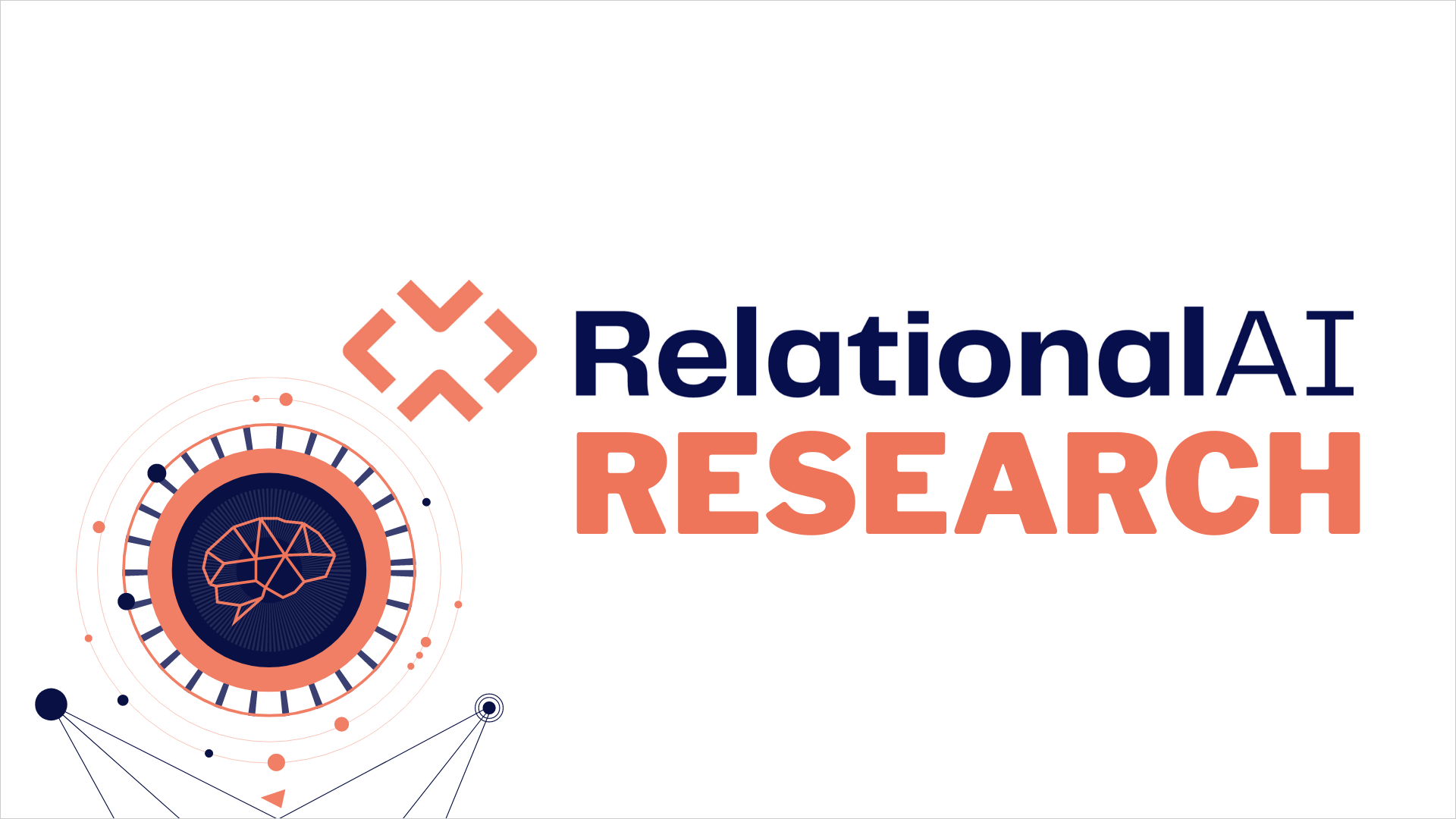
Product Collection Recommendation in Online Retail
Recommender systems are an integral part of eCommerce services, helping to optimize revenue and user satisfaction. Bundle recommendation has recently gained attention by the research community since behavioral data supports that users often buy more than one product in a single transaction. In most cases, bundle recommendations are of the form “users who bought product A also bought products B, C, and D”. Although such recommendations can be useful, there is no guarantee that products A,B,C, and D may actually be related to each other. In this paper, we address the problem of collection recommendation, i.e., recommending a collection of products that share a common theme and can potentially be purchased together in a single transaction.
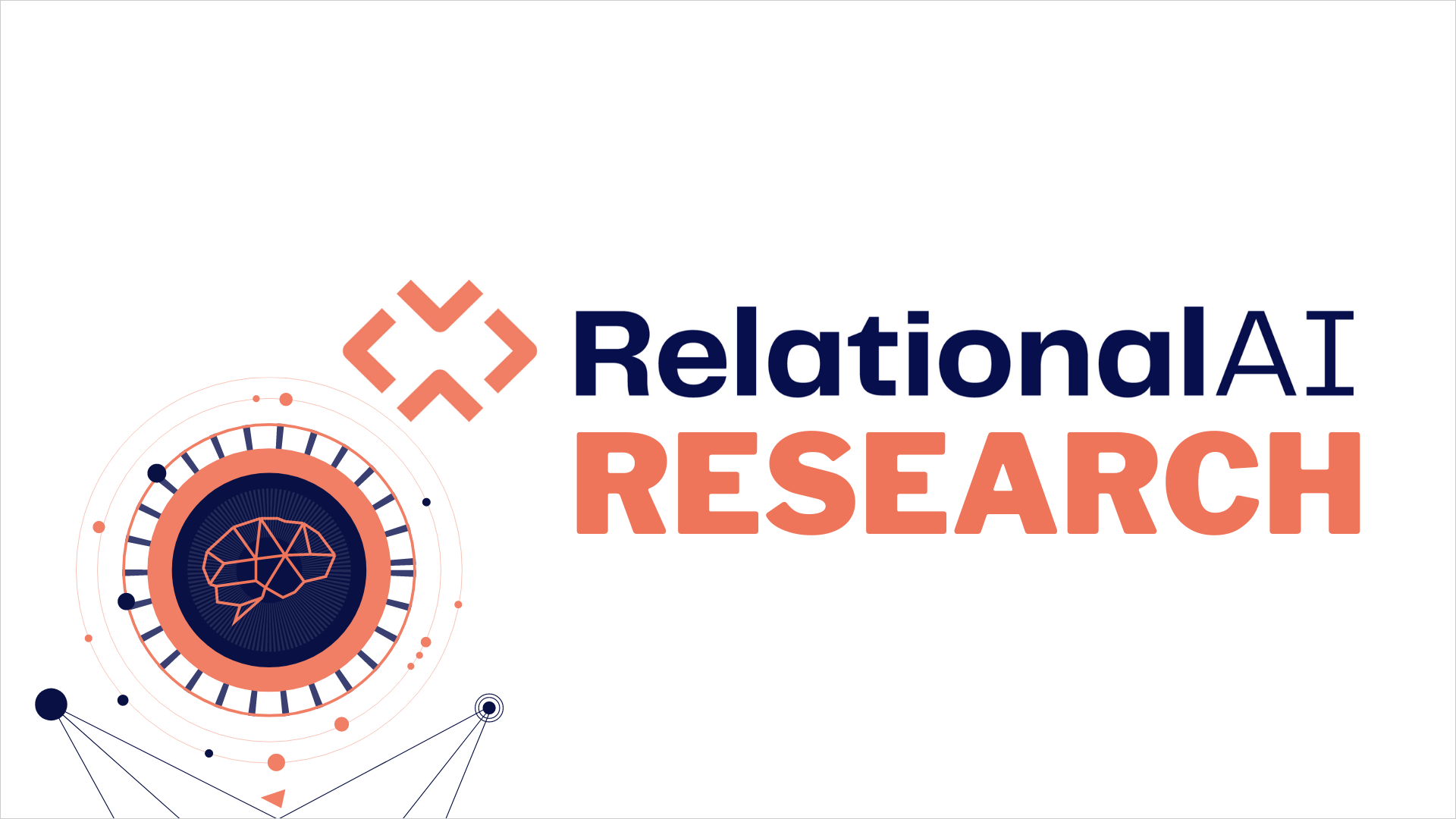
Algebraic Modeling in Datalog
Datalog is a deductive language tailored for easy database access. We introduce an algebraic modeling language in Datalog for mixed-integer linear optimization models.
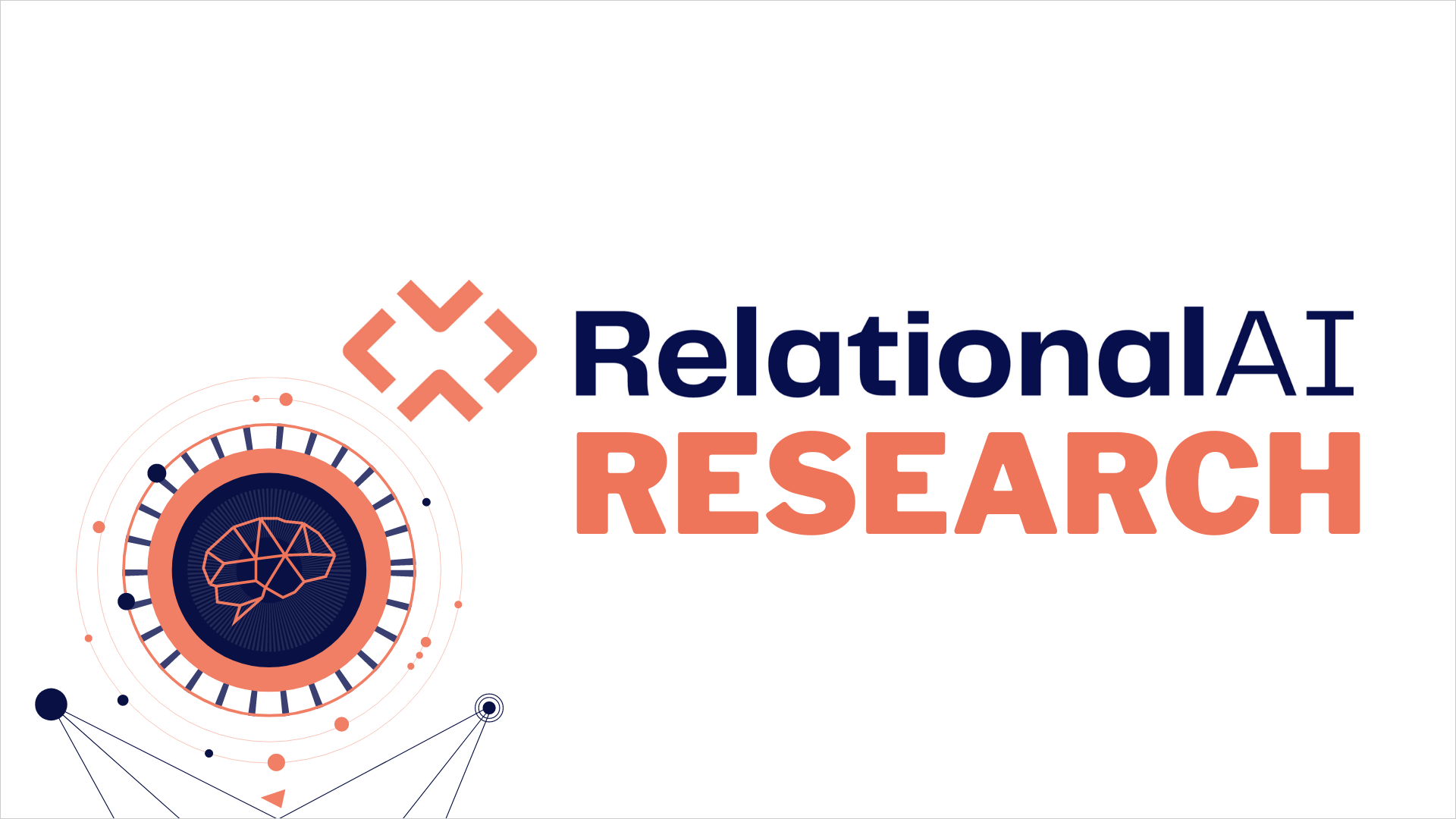
Rk-means: Fast Clustering for Relational Data
This RelationalAI Research paper introduces Rk-means, or relationalk-means algorithm, for clustering relational data tuples without having to access the full data matrix.
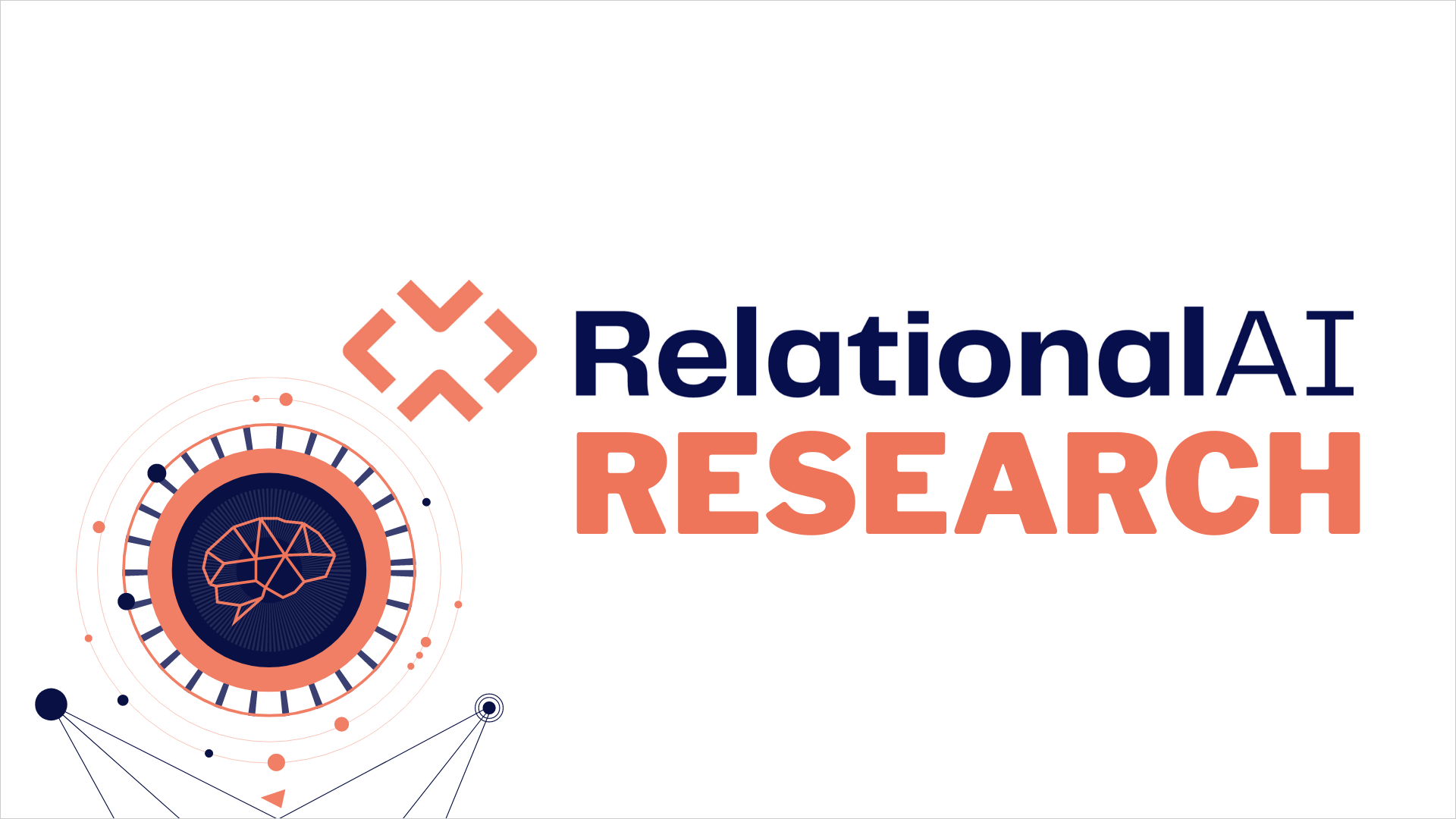
Defensive Points-To Analysis: Effective Soundness via Laziness
In this work, we present a more principled approach for identifying precision-critical methods, based on general patterns of value flows that explain where most of the imprecision arises in context-insensitive pointer analysis.
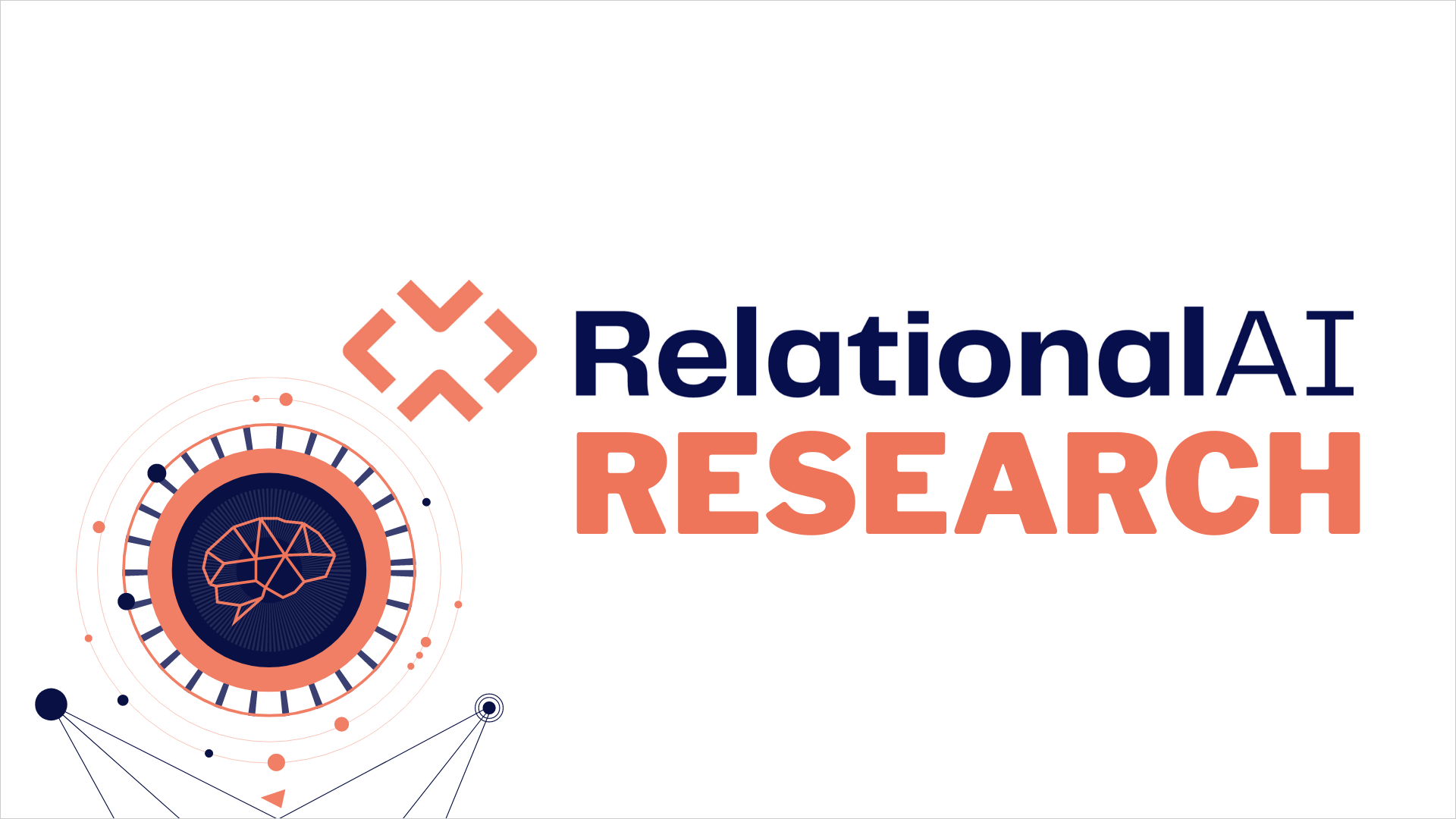
Worst-Case Optimal Join Algorithms: Techniques, Results and Open Problems
Worst-case optimal join algorithms are the class of join algorithms whose runtime match the worst-case output size of a given join query. While the first provably worst-case optimal join algorithm was discovered relatively recently, the techniques and results surrounding these algorithms grow out of decades of research from a wide range of areas, intimately connecting graph theory, algorithms, information theory, constraint satisfaction, database theory, and geometric inequalities.